Technological advances have always opened up new vistas for storytelling and marketing. Just like the introduction of TV ushered in an era of mass advertising and reach never achieved before, the arrival of the internet and mobile subsequently, brought in a totally new level of targeting and contextual advertising. AI is now all set to change how people interact with technology, information, brands and services.
Let's take a quick peek at some of the AI applications currently on in marketing and advertising:
- AI generated content
- Smart content creation
- Voice search
- Programmatic media buying
- Propensity modelling
- Predictive analysis
- Ad targeting
- Dynamic Pricing
- Retargeting
- Predictive customer service
Given below is a RACE (Reach, Act, Convert, Engage) machine learning customer life cycle infographic to show how AI, Machine Learning and Propensity Modelling can be applied to different customers. This article assumes that the reader is familiar with the basics of AI and Machine Learning (For a quick primer, look up Understanding Artificial Intelligence and the Shades of Difference Among its Subsets ). A Propensity Model is a statistical scorecard to predict the behaviour of the customer or the prospect base. This could be typically used to predict those who are most likely to respond to an offer or to focus retention activity on those most likely to churn.
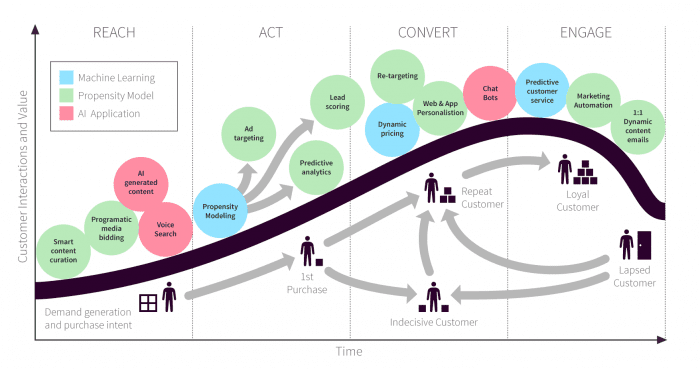
Let's now look at some of the applications more in detail:
Programmatic Advertising:
Programmatic Advertising is the automated process of buying and selling ad inventory through an exchange which connects advertisers to publishers. This process uses AI technologies & real-time bidding for inventory across mobile, display, video and social channels – even making its way into television. AI technologies have algorithms that analyze a visitor’s behaviour allowing for real time campaign optimizations towards an audience more likely to convert. Programmatic companies have the ability to gather this audience data to then target, more precisely, whether it’s from the 1st party (their own) or from a 3rd party data provider.
Ad Targeting:
One of the greatest revenue growth opportunities for both publishers and media resellers today are targeted impressions or ads. These targeted ads drive revenue through two distinct ways:
a) Higher prices: Advertisers are willing to spend a significantly higher amount for targeted advertising. Prices can increase by as much as 50% if targeting is included.
b) Higher volume: Targeted campaigns are far more attractive to advertisers and brands, which translates to them running more of these.
AI and machine learning algorithms are used to compute which user belongs to which audience. Prediction accuracy is usually quite good.
Retargeting:
Retargeting is the practice of serving ads based on prior engagement. While there is more than one form of this technology, the most frequently used is site-based retargeting.
Changes are controlled by pricing bots, which are software agents that gather data and use algorithms to adjust pricing according to business rules. Typically, the business rules take into account such things as the customer's location, the time of day, the day of the week, the level of demand and competitors' pricing. Big data analytics enables the business rules for price adjustments to become a lot more granular. By collecting and analyzing data about a particular customer, a vendor can more accurately predict what price the customer is willing to pay and adjust prices accordingly.
Site-based retargeting uses cookies to stay in front of previous site visitors. When someone visits your website, a few lines of code provided by your retargeter will drop an anonymous browser cookie. This cookie is a small file that stores information. The cookie will store the site visit, but does not store any sensitive information which could identify the visitor. The cookie lets your retargeter know when one of your bounced visitors appears on another site. If there is available ad space, your retargeter will bid on that space in real-time, and if they are the highest bidder, will secure the ad space before the page loads. This entire process is automated and occurs within a fraction of a second.
Dynamic Pricing:
Dynamic pricing, also known as real-time pricing, is an approach to setting the cost for a product or service that is highly flexible. The goal of dynamic pricing is to allow a company that sells goods or services over the Internet to adjust prices dynamically in response to actual and projected market demands.
Changes are controlled by pricing bots, which are software agents that gather data and use algorithms to adjust pricing according to business rules. Typically, the business rules take into account such things as the customer's location, the time of day, the day of the week, the level of demand and competitors' pricing. Big data analytics enables the business rules for price adjustments to become a lot more granular. By collecting and analyzing data about a particular customer, a vendor can more accurately predict what price the customer is willing to pay and adjust prices accordingly.
Marketing Forecasting:
One of the most straightforward usages of business intelligence data is its ability to aid in predictions, a capability further enhanced by AI. Because of the high volume and generally quantifiable nature of marketing data like clicks, views, time-spent-on-page, email responses and purchases, machine learning models can be trained far more quickly on marketing data compared to data available in other functional domains.
Image recognition / Machine vision:
Soon, it will be possible for consumers to search for a specific product, or similar products, by pulling up an image. This could be as simple as snapping a photo of a pair of shoes that one is keen to buy or using a web app to select a certain image that one found, say on Google, using 'Search'.
Content Generation:
Already, a significant number of sports and finance-related articles are written by machines, not by humans. Companies have found ways to turn specific sets of information (domains like sports and finance are laden with time data and numbered data) into articles which can be easily read by humans and are mostly indistinguishable from those written by human beings. In the future, companies may have product descriptions and entire product line-related articles composed entirely by machines, based on information about the products in question. Personalization of content will also be an important future trend.
Recommendation Engine:
Recommendations Engines - one of the concepts in AI - is fast gaining momentum. It is a perfect marketer tool especially for e-commerce / online businesses and is very useful to increase turnarounds. Recommendation Engines (also known as Recommender Systems) started off becoming popular in the retail industry, typically in the online retail & e-commerce space for personalized product recommendations. Possibly the most common and easily understood usage is Amazon’s section on 'Customers who bought this item also bought …'. It is akin to a smart and intelligent salesperson who knows the customer's taste and preferences and is thus able to make smart decisions about what recommendations would be appealing to the customer most, thus increasing significantly the possibility of a conversion. Though it originally started off in e-commerce, it is now gaining popularity in other sectors, especially in Media.
Recommendations Engines - one of the concepts in AI - is fast gaining momentum. It is a perfect marketer tool especially for e-commerce / online businesses and is very useful to increase turnarounds. Recommendation Engines (also known as Recommender Systems) started off becoming popular in the retail industry, typically in the online retail & e-commerce space for personalized product recommendations. Possibly the most common and easily understood usage is Amazon’s section on 'Customers who bought this item also bought …'. It is akin to a smart and intelligent salesperson who knows the customer's taste and preferences and is thus able to make smart decisions about what recommendations would be appealing to the customer most, thus increasing significantly the possibility of a conversion. Though it originally started off in e-commerce, it is now gaining popularity in other sectors, especially in Media.
Predictive Analytics for Marketing:
Today, well over 30 years after electronic spreadsheets first saw the light of day, medium-sized businesses are often still operating their marketing “scoreboards” in spreadsheets. But businesses with an eye on the future want to know more than just what happened in the past. Most analytics tools and tracking don’t quite tell you what the score will be. More and more executives are searching for ways to look forward with their numbers, not just back. That's where predictive analytics comes in.
Predictive analytics is the use of data, statistical algorithms and machine learning techniques to identify the likelihood of future outcomes based on historical data. The goal is to go beyond knowing what has happened to providing a best assessment of what will happen in the future.
In the 21st century economy, a marketing unit without machine learning mastery operates at a serious handicap. However, adopting an ML solution without understanding what it truly does, can do more harm in terms of wasted hours and dollars than good. Machine Learning is not magic and won’t automatically move the needle unless your team selects and configures the right AI solution for specific marketing challenges. The problem is, some “experts” sell AI products like snake oil, promising an elixir to cure all your marketing woes. Managers and marketing professionals need to clearly identify their business goals and metrics of success before evaluating AI and automation solutions.
Comments
Post a Comment