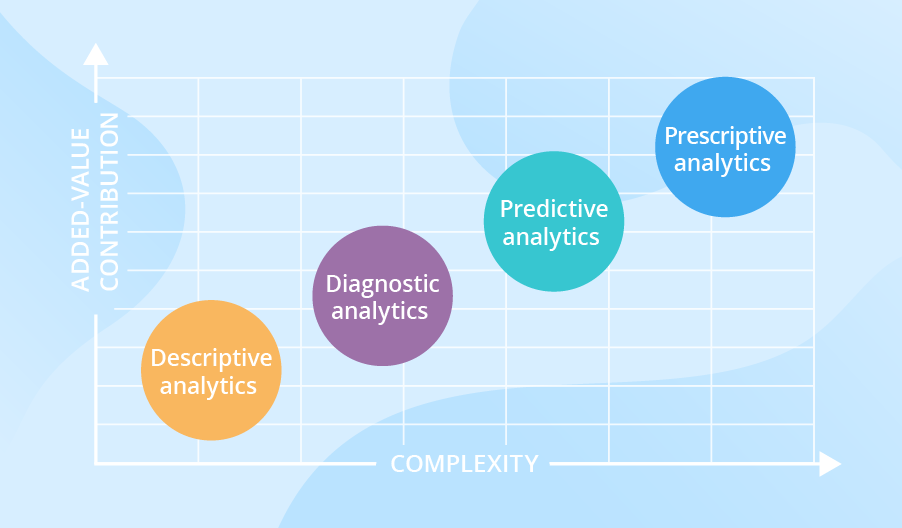
Businesses have been using analytics of one kind or another, for a very long time now. Even before manual spreadsheets, back-of-the-envelope computations and intuitive analytics were resorted to frequently to understand what happened in the past and to draw some lessons from that understanding. The advent of computers and apps like electronic spreadsheets really provided a whole new fillip to the domain of analytics. The availability of huge amounts of data, computing power including distributed and cloud computing and various tools to filter, churn and massage the data to find patterns otherwise difficult to discern, led to the boom in predictive and prescriptive analytics in addition to the classical descriptive analytics.
In simple terms, descriptive analytics is the use of historical data to better understand changes that have occurred in a business and to enter into various comparisons as may be necessary. Predictive analytics is a branch of advanced analytics which uses techniques like data mining, modelling and machine learning among others to make more accurate and reliable forecasts and projections about the future. Prescriptive analytics draws on the outcomes generated by descriptive and / or predictive analytics to suggest the optimal action or strategy. The time frames for these recommended actions or strategies could be anywhere from immediate to long-term in the future.
Big Data Analytics typically refers to the process of using large volume and varied datasets, both structured and unstructured, to uncover information like hidden patterns and trends, correlations and customer preferences. Depending on the desired outcome, analytics, particularly predictive and prescriptive analytics, could both use big data.
Having covered the basics, let us now get down to a few use cases of prescriptive analytics:
Prescriptive Analytics in Healthcare:
Prescriptive Analytics offers healthcare decision makers the window for optimal future outcomes. Based on decision optimization technology, this would enable users to not only recommend the best course of action for patients or providers, but would also enable them to compare multiple what-if scenarios to assess the impact of choosing one action over another.
Let us consider a hypothetical example: a health insurance organization spots a pattern in its claims data for the previous year which shows that a significant percentage of its diabetic patients also suffer from retinopathy. Using predictive analytics, the insurance company then estimates the probability of an increase in ophthalmology claims during the next plan year. Subsequently, prescriptive analytics is used to model out the cost impact if average ophthalmology reimbursement rates remain the same, increase or decrease during the next plan year. Based on this modelling, an optimal course of action is recommended.
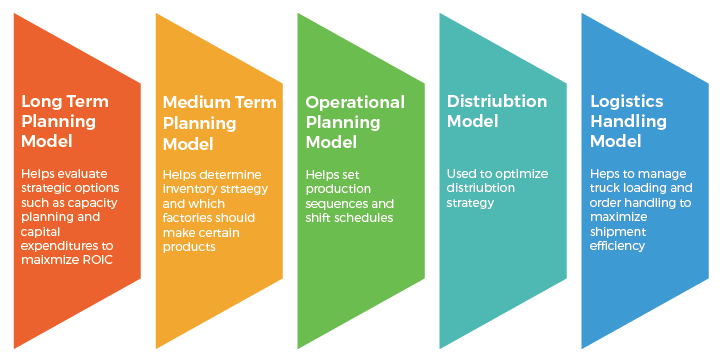
Prescriptive Analytics in Manufacturing:
Keeping in view profit maximization, manufacturers need to be able to respond to market demand with optimal production cost, speed and flexibility. The problem may be defined as determining how much of each product to produce, when to produce it and in which location. Significant fluctuations in demand can occur due to seasonal trends and other factors. Since factories have limited production resources, the challenge lies in considering all constraints to maximize use of available equipment and personnel, minimize product costs and downtime, and deliver orders within the time frames committed. To achieve this, several manufacturers rely on decision optimization solutions to find the best possible ways to make use of their available materials, staff and machine capacity.
Prescriptive Analytics in Marketing:
Prescriptive analytics can be particularly useful for marketing mix modelling. Let us take the example of a marketer wanting to optimize the mix of paid advertising across various channels like Google AdWords, Facebook, Twitter, LinkedIn, into different audiences, with different messages. Since these channels each report on cost per click/impression over time, that data can be leveraged in a manufacturing paradigm as a resource with a specific variable cost and yield, based on clicks registered, over time. A good marketer will understand how those clicks translate into revenue which ultimately allows the company to optimize the advertising spend so as to maximize the revenue for that spend.
Comments
Post a Comment